QM9 example
This example will be used to highlight some of the more advanced features of the
Dataset class using the popular QM9 dataset.
It is suggested that you go through the basic example first. The complete
code will not be shown in this example (for the complete code, see the Jupyter
notebook at colabfit/examples/QM9/qm9.ipynb
); instead, only the additional features will be
discussed here.
Note that this example assumes that the raw data has already been downloaded using the following commands:
$ mkdir qm9
$ cd qm9 && wget -O dsgdb9nsd.xyz.tar.bz2 https://figshare.com/ndownloader/files/3195389 && tar -xvjf dsgdb9nsd.xyz.tar.bz2
Writing a custom property for QM9
The QM9 dataset contains a large number of computed properties for each Configuration, as documented in its original README.
In order to preserve this information, a custom property can be defined (see Property definitions for more information).
client.insert_property_definition({
'property-id': 'qm9-property',
'property-title': 'A, B, C, mu, alpha, homo, lumo, gap, r2, zpve, U0, U, H, G, Cv',
'property-description': 'Geometries minimal in energy, corresponding harmonic frequencies, dipole moments, polarizabilities, along with energies, enthalpies, and free energies of atomization',
'a': {'type': 'float', 'has-unit': True, 'extent': [], 'required': True, 'description': 'Rotational constant A'},
'b': {'type': 'float', 'has-unit': True, 'extent': [], 'required': True, 'description': 'Rotational constant B'},
'c': {'type': 'float', 'has-unit': True, 'extent': [], 'required': True, 'description': 'Rotational constant C'},
'mu': {'type': 'float', 'has-unit': True, 'extent': [], 'required': True, 'description': 'Dipole moment'},
'alpha': {'type': 'float', 'has-unit': True, 'extent': [], 'required': True, 'description': 'Isotropic polarizability'},
'homo': {'type': 'float', 'has-unit': True, 'extent': [], 'required': True, 'description': 'Energy of Highest occupied molecular orbital (HOMO)'},
'lumo': {'type': 'float', 'has-unit': True, 'extent': [], 'required': True, 'description': 'Energy of Lowest occupied molecular orbital (LUMO)'},
'gap': {'type': 'float', 'has-unit': True, 'extent': [], 'required': True, 'description': 'Gap, difference between LUMO and HOMO'},
'r2': {'type': 'float', 'has-unit': True, 'extent': [], 'required': True, 'description': 'Electronic spatial extent'},
'zpve': {'type': 'float', 'has-unit': True, 'extent': [], 'required': True, 'description': 'Zero point vibrational energy'},
'u0': {'type': 'float', 'has-unit': True, 'extent': [], 'required': True, 'description': 'Internal energy at 0 K'},
'u': {'type': 'float', 'has-unit': True, 'extent': [], 'required': True, 'description': 'Internal energy at 298.15 K'},
'h': {'type': 'float', 'has-unit': True, 'extent': [], 'required': True, 'description': 'Enthalpy at 298.15 K'},
'g': {'type': 'float', 'has-unit': True, 'extent': [], 'required': True, 'description': 'Free energy at 298.15 K'},
'cv': {'type': 'float', 'has-unit': True, 'extent': [], 'required': True, 'description': 'Heat capacity at 298.15 K'},
'smiles-relaxed': {'type': 'string', 'has-unit': False, 'extent': [], 'required': True, 'description': 'SMILES for relaxed geometry'},
'inchi-relaxed': {'type': 'string', 'has-unit': False, 'extent': [], 'required': True, 'description': 'InChI for relaxed geometry'},
})
Note that a property definition is used for performing verification checks when
parsing the data. A property_map
must still be provided for specifying
how to parse the data and what the units of the fields are.
property_map = {
'qm9-property': {
# Property Definition field: {'field': ASE field, 'units': ASE-readable units}
'a': {'field': 'A', 'units': 'GHz'},
'b': {'field': 'B', 'units': 'GHz'},
'c': {'field': 'C', 'units': 'GHz'},
'mu': {'field': 'mu', 'units': 'Debye'},
'alpha': {'field': 'alpha', 'units': 'Bohr*Bohr*Bohr'},
'homo': {'field': 'homo', 'units': 'Hartree'},
'lumo': {'field': 'lumo', 'units': 'Hartree'},
'gap': {'field': 'gap', 'units': 'Hartree'},
'r2': {'field': 'r2', 'units': 'Bohr*Bohr'},
'zpve': {'field': 'zpve', 'units': 'Hartree'},
'u0': {'field': 'U0', 'units': 'Hartree'},
'u': {'field': 'U', 'units': 'Hartree'},
'h': {'field': 'H', 'units': 'Hartree'},
'g': {'field': 'G', 'units': 'Hartree'},
'cv': {'field': 'Cv', 'units': 'cal/mol/K'},
'smiles-relaxed': {'field': 'SMILES_relaxed', 'units': None},
'inchi-relaxed': {'field': 'SMILES_relaxed', 'units': None},
}
}
Defining a reader function
Since the data in QM9 is not stored in a typical format (it uses an uncommon
modification to the typical XYZ format), it is necessary to use the
FolderConverter
class, with a custom
reader()
function.
def reader(file_path):
# A function for returning a list of ASE a
properties_order = [
'tag', 'index', 'A', 'B', 'C', 'mu', 'alpha', 'homo', 'lumo', 'gap', 'r2', 'zpve', 'U0', 'U', 'H', 'G', 'Cv'
]
with open(file_path, 'r') as f:
lines = [_.strip() for _ in f.readlines()]
na = int(lines[0])
properties = lines[1].split()
symbols = []
positions = []
partial_charges = []
for line in lines[2:2+na]:
split = line.split()
split = [_.replace('*^', 'e') for _ in split] # Python-readable scientific notation
# Line order: symbol, x, y, z, charge
symbols.append(split[0])
positions.append(split[1:4])
partial_charges.append(split[-1])
positions = np.array(positions)
partial_charges = np.array(partial_charges, dtype=float)
atoms = Atoms(symbols=symbols, positions=positions)
atoms.info['mulliken_partial_charges'] = partial_charges
name = os.path.splitext(os.path.split(file_path)[-1])[0]
atoms.info['name'] = name
for pname, val in zip(properties_order[2:], properties[2:]):
atoms.info[pname] = float(val)
frequencies = np.array(lines[-3].split(), dtype=float)
atoms.info['frequencies'] = frequencies
smiles = lines[-2].split()
inchi = lines[-1].split()
atoms.info['SMILES'] = smiles[0]
atoms.info['SMILES_relaxed'] = smiles[1]
atoms.info['InChI'] = inchi[0]
atoms.info['InChI_relaxed'] = inchi[1]
yield atoms
dataset.configurations = load_data(
file_path='qm9',
file_format='folder',
name_field='name', # key in Configuration.info to use as the Configuration name
elements=['H', 'C', 'N', 'O', 'F'], # order matters for CFG files, but not others
default_name='qm9', # default name with `name_field` not found
reader=reader,
glob_string='*.xyz',
verbose=True
)
from colabfit.tools.property_settings import PropertySettings
pso = PropertySettings(
method='DFT/B3LYP/6-31G(2df,p)',
description='QM9 property settings calculation',
files=None,
labels=['DFT', 'B3LYP', '6-31G(2df,p)'],
)
ids = list(client.insert_data(
images,
property_map=property_map,
property_settings={'qm9-property': pso},
generator=False,
verbose=True
))
Cleaning the dataset
Using the plot_histograms()
and
get_statistics()
functions reveals that
the QM9 dataset has some outlying data entries.
client.get_statistics(
['qm9-property.a', 'qm9-property.b', 'qm9-property.c'],
ids=dataset.property_ids,
verbose=True
)
# 'qm9-property.a': {'average': 9.814382088508797, 'std': 1809.4589082320583, 'min': 0.0, 'max': 619867.68314, 'average_abs': 9.814382088508797}
# 'qm9-property.b': {'average': 1.4060972645920002, 'std': 1.5837889998648804, 'min': 0.33712, 'max': 437.90386, 'average_abs': 1.4060972645920002}
# 'qm9-property.c': {'average': 1.1249210272988013, 'std': 1.0956136904779634, 'min': 0.33118, 'max': 282.94545, 'average_abs': 1.1249210272988013}
client.plot_histograms(
['qm9-property.a', 'qm9-property.b', 'qm9-property.c',],
ids=dataset.property_ids
)
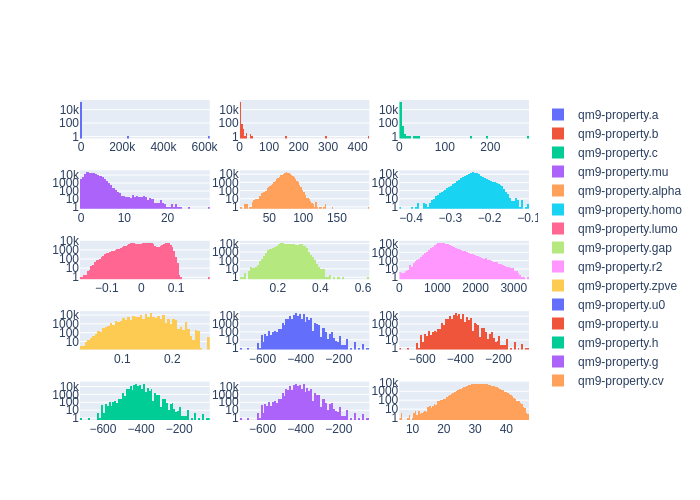
The filter_on_properties()
function can be used to filter on the ConfigurationSets and Properties.
clean_config_sets, clean_property_ids = client.filter_on_properties(
ds_id=ds_id,
filter_fxn=lambda x: (x['qm9-property']['a']['source-value'] < 20) and x['qm9-property']['b']['source-value'] < 10,
fields=['qm9-property.a.source-value', 'qm9-property.b.source-value'],
verbose=True
)
new_cs_ids = []
for cs in clean_config_sets:
new_cs_ids.append(
client.insert_configuration_set(
cs.configuration_ids,
cs.description, verbose=True
)
)
ds_id_clean = client.insert_dataset(
cs_ids=new_cs_ids,
pr_ids=clean_property_ids,
name='QM9_filtered',
authors=dataset.authors,
links=[
'https://www.nature.com/articles/sdata201422',
'https://figshare.com/collections/Quantum_chemistry_structures_and_properties_of_134_kilo_molecules/978904'
],
description="The QM9 dataset, filtered by removing values with a>=20 or b>=10",
resync=True,
verbose=True,
)
client.plot_histograms(
['qm9-property.a', 'qm9-property.b', 'qm9-property.c',],
ids=dataset.property_ids
)
client.get_statistics(
['qm9-property.a', 'qm9-property.b', 'qm9-property.c'],
ids=dataset.property_ids,
verbose=True
)
# 'qm9-property.a': {'average': 3.407053427070018, 'std': 1.3368223663235594, 'min': 0.0, 'max': 19.99697, 'average_abs': 3.407053427070018}
# 'qm9-property.b': {'average': 1.3966863945821093, 'std': 0.45813797072575396, 'min': 0.33712, 'max': 9.93509, 'average_abs': 1.3966863945821093}
# 'qm9-property.c': {'average': 1.1177706236464617, 'std': 0.328798457356026, 'min': 0.33118, 'max': 6.46247, 'average_abs': 1.1177706236464617}
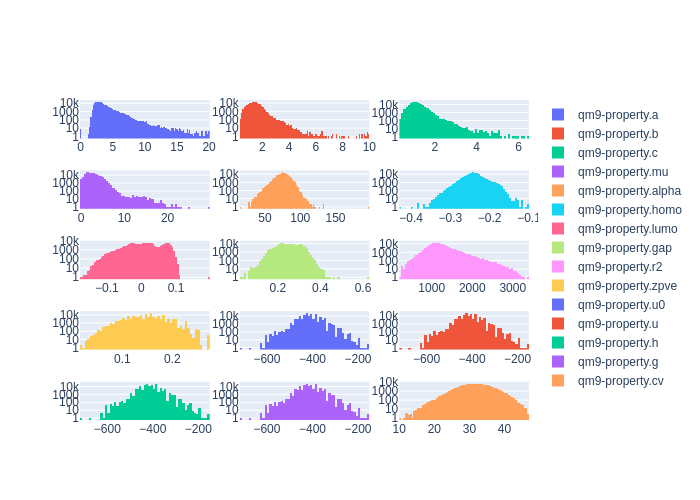